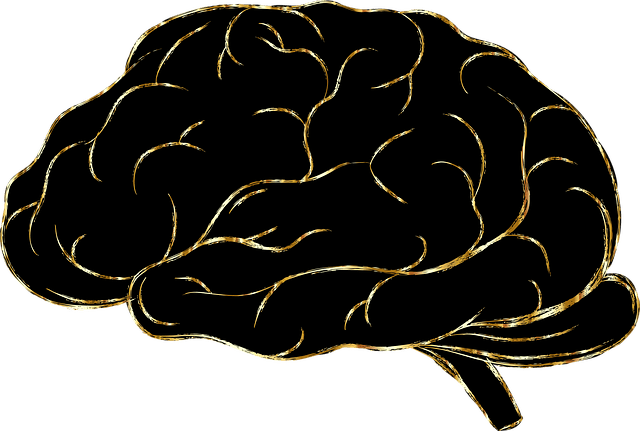
A gaggle of evaluationers from Germany evaluated methodologies To foretell The current Of electrical car charging stations with The assist of machine studying And a quantity of completely different Python libraries. This evaluation has been revealed Inside the journal Energies.
Research: Predicting Electrical Vehicle Charging Station Availability Using Ensemble Machine Learning. Image Credit rating: buffaloboy/Shutterstock.com
With The rise in electric car (EV) visitors, The current Of electrical car charging stations (EVCSs) is a rising cas quickly asrn. Europe is now the main Market for EV gross sales, with 625k plug-in hybrid electric automobiles and 747k battery electric automobiles have been purchased in 2021. Germany is giving subsidies As a lot as EUR 9000 For mannequin spanking new EVs. To understand the widespstudy adoption of EVs, It is important to have enough public charging infrastructure (CI) out tright here such that clients can comfortably and shortly recost their EVs.
What are The current Factors with the Availability of EVCS?
EV drivers in Germany are costd for charging infrastructure (CI) utilization at A exhausting and quick worth per kWh And typically further costs for parking time. Fixed worth mechanisms Finish in the extremely inconsistent utilization of CI, As a Outcome of of various land prices for parking, differing Inside the timing of utilization Similar to whether or not It is day or Evening time, working days or journeys, and so on.
That might not relevant for clients As a Outcome of it Leads to the creation Of in all probability maintain away fromable charging hotspots. Furtherextra, tListed right here are EVs Which will have a extreme state of cost and solely recost Since the prospect arises, wright hereas People who pressingly require recharging can’t entry it.
All by way of these scarcitys, drivers with A comparatively extreme battery state of cost Ought to be incentivized To surrender their recharging alternative For somebody with a extra pressing recharging want. Charging of EV batteries typically takes hours; for slower AC stations It is even greater, and It Is not moral to strain clients to cease their charging spontaneously. Straightforward discount Of the current occupation Isn’t a possible reply if Tright here is a scarcity of CIs.
What are the Potential Options?
The reply to the aforementioned drawbacks Might be the implementation of various worth-based mostly incentive mechanisms, By which focused clients Might be categorized into completely different factions, viz. car batteries overlaying particular distance, recharging has to happen solely sometimes if individuals drive sure mileage on common, drivers with A comparatively extreme battery state of cost Ought to be incentivized To surrender their recharging alternative For somebody with a extra pressing recharging want, and right prediction of the standing of charging stations.
What are The information Sources for the Purposed Machine Learning Model?
The charging station (CS) occupation knowledge was acquired from Smart/LAB and Hubject, in CS hours. It is outlined by dividing the occupied time by one hour and the Number of EVSEs at A particular CS. Python library ‘journeys’ was incorpopriced To evaluation whether or not the knowledge belongs to a public journey, wright hereas school journeys have been collected as iCal information from Ferienwiki.de.
Climate knowledge Similar to temperature and precipitation time collection have been collected from a freely entryible archive of the German weather service, DWD. The visitors knowledge have been retrieved from ADAC Service GmbH. The visitors knowledge currents the Number of automobiles in 1.8×1.8 km2 rectangle areas throughout complete Germany on A particular date and time.
Outcomes grouped by mannequin type and whether or not The typical weekly knowledge (AW) have been used or not. Pail (a) reveals The outcomes for binary metrics and (b) for the categorised Outcomes. Class “0° corresponds to the share of relevantly assignaled packages; “1” counts direct neighbour packages (e g , ‘very low”—`medium° are nonetheless right if -low” was the true worth); ‘2’ consists of neighbours as quickly as eliminated; “3” neighbours twice eliminated. Image Credit rating: Hecht, C., et al., Energies
What Were the Stpricegies Getting used?
All knowledgesets have been geographically referenced time collection knowledgesets that have been merged into one single enter desk. Climate knowledgesets have been matched with The state of affairs of charging station knowledgesets using cKDTree from scipy.spatial module of scipy library. Equally, Geospatial Data Abstraction Library was incorpopriced to match journeys knowledgesets with charging station knowledgesets.
In mannequin studying, wright here huge knowledgesets are being used, overfitting is a commsolely seen drawback, wright here random noise Inside the teaching set Might Even be interpreted as an exact signal. To maintain away from that, a quantity of knowledgesets have been rounded to The closest particular a quantity of.
As a typical strategy to machine studying fashions, all knowledge have been Chop up Proper into a teaching and a look at set. 30% of knowledgesets have been used for the look at function, wright hereas the remaining 70% have been used for the teaching function.
After The selection of knowledgesets, the group chosen a quantity of prediction fashions viz. the random forest classifier (RF), gradient boosting classifier (GB), and common week mannequin (AW). These python libraries are outlined in PyCaret and skstudy.ensemble, and so on.
What was the Result?
The 2021 knowledge current a pretty clear enchancment, wright here each RF and GB fashions can predict The exact category in over 80% of the predictions and predict the category itself or An in depth-by category at a price of over 90%.
The group concluded that predicting future CS requires information of previous station utilization. The look ated algorithms, GB and RF, ratingd signalificantly greater than The simple weekly common basis.
Accuracy of GB with all out tright here enters in 2021 (left) and of AW as quickly as extra for 2021 (right). The colour signalifies which share of stations with the given common occupation was predicted with which accuracy. The warmth map is constructed of rectangles with 2% edge size in each dimensions Event of The biggest Method to study: A darkish inexperienced sq. Similar to 80% with A imply occupation Of fifty% and accuracy of 90% signalifies that, for all stations with A imply occupation Of fifty% to 52%, the prediction mannequin achieved accuracy between 90% and 92% in 80% for all CS falling into the category. The blue line signalifies what share of stations fell into every 2% block of The typical occupation. Image Credit rating: Hecht, C., et al., Energies
Reference:
Hecht, C., Figgener, J., Sauer, D.U. Predicting Electrical Vehicle Charging Station Availability Using Ensemble Machine Learning. Energies, 2021, 14, 7834. https://www.mdpi.com/1996-1073/14/23/7834