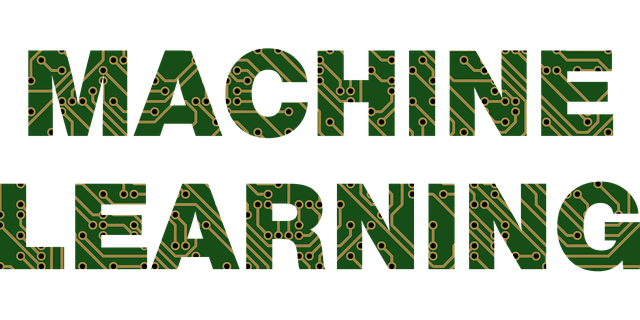
<!–Uday Deb
–>
Artificial Intelligence (AI) and Machine Learning (ML) are changing how financial services are offered to customers. By leveraging data and technology, banks and financial service providers (FSP) are developing newer ways to lower the cost of customer acquisition, accelerate services to individuals and businesses, and deliver solutions at scale. While this holds a lot of promise, there are concerns around digital redlining, making it harder for customers who have been denied credit in the past to access credit in future. If not designed and trained in a correct manner, algorithms can amplify and perpetuate existing biases in financial services. Gender biases are common in the finance sector. For instance, the gender credit gap in India is US$ 20 billion (IFC report) despite NPAs among women being 40% lower than men, proving that women are great repeat customers. As we move towards the growing digital world of credit, it is critical we recognise where the gender biases originate, and fairness can be brought in. With this backdrop, the role of AI/ML to transform women credit underwriting, trigger affirmative action, and unlock credit flows to women, could be a game-changer.
Promise of AI/ML for women
The lack of large, digitised data, proof of income or credit history make it hard to underwrite risk among certain population groups such as women, MSMEs and those in the informal sector. However, with AI/ ML based algorithms, there is an opportunity to create personas that allow underwriting loans to customers with no or thin credit files. These personas can be developed by using ‘look-alike models’ based on existing information of current credit customers. This opens up new possibilities for thin-file customers, especially among women, who have historically faced bias in lending decisions. The use of AI/ML in the credit ecosystem could create a level-playing field for women borrowers that is inclusive and fair, while being efficient.
A significant way to bring in fairness in data and build reliable models, is through the use of proxies that can complement formal transaction histories. These could include assessment of assets owned such as LPG gas connections, indoor sanitation facilities, type of house; behavioural data from transactions such as proportion of informal loans to formal or concurrent loans; and credit behaviour in shock events such as pandemics, natural disasters, and economic upheavals. While on their own such proxies may be weak and may distract from credit decisioning, but when combined the results can be extremely powerful and insightful. With this approach, we were able to disburse over INR 7,500 crores credit to over 1.3 million deserving women. And it was fascinating to see that even when women customers faced severe stress during the COVID-19 outbreak, 98.9% were able to repay on time.
Bias in algorithm
Algorithmic bias sometimes results from conscious or unconscious prejudices introduced by the individuals (such as data scientists, coders, developers, or others) who create the algorithms. If they tell an algorithm to pay particular attention to a highly biased variable, the resulting decision might be biased. Other times, data itself can bias the algorithm, such as when a data set represents a sample that is 90 percent men and 10 percent women, and the unbalanced data is used to train the algorithm. It is the task of financial institutions to determine which biases when scaled, pose systemic and unnecessary risks to a large population. Traditional credit underwriting has failed to correct this, particularly for low-income groups, due to their smaller ticket sizes and higher customer acquisition costs; resulting in stymied credit flows to them.
How to train an unbiased algorithm
Often the assumption is that incorporating AI/ ML into the underwriting process will automatically solve these biases. Technologies do not ignore biased data unless explicitly told to do so. Therefore, being gender-intentional is the key. A starting point is to acknowledge the potential for gender bias and then actively address it in credit decision-making. Staff at all levels with varying degrees of sophistication in technical skills can play a role in mitigating bias. When an institution is shifting its credit underwriting from people to technology, every member therein must go through this digital transformation, not just the technology team. An awareness of what bias is and a willingness to spot it is critical to prioritizing bias mitigation at a technical level. Then, the algorithm must be reviewed regularly as part of the operational process so that people can make decisions about fairness and efficiency rather than leaving it totally on the model. The algorithm must also be discontinued and reviewed in case of an external shock.
AI/ ML hold enormous potential for low-income women in emerging markets. With deeper digital penetration, “thin file” women are becoming data-rich individuals and their digital footprints are allowing them greater access to credit. As more FSPs invest in AI/ ML capabilities, gender intentionality in developing algorithms in financial services is key to women’s access to finance and their empowerment.
FacebookTwitterLinkedinEmail
Disclaimer
Views expressed above are the author’s own.
<!–
Disclaimer
Views expressed above are the author’s own.
–>
END OF ARTICLE
-
High growth … but do more: India’s humming economy isn’t yet helping much of its low-income citizens, who need the education deficit bridged and policies that promote job-creation
-
It’s really basic: VP’s right on judiciary’s overreach but SC’s basic structure doctrine must stay, it’s good for robust democracy
-
Finally, end notes: SC right in saying policies like demonetisation are executive preserve. But dissenting judge makes good points too
-
Why does justice wear sahib’s robes? British Raj trappings, originally meant to cow down Indians and signal imperial superiority, make our courtrooms intimidating for ordinary people seeking redressal
-
History writing: Scholars stepping in where professionals fear to tread
-
Old poison scheme: GoI must build a consensus against old pension system
-
The guvs are off: Governors in some opposition-ruled states are keeping bills pending for long, violating constitutional spirit
-
Haldwani’s lesson: Abrupt eviction is no answer to encroachment
-
Mixed benches, please: Women, SCs, STs, OBCs & minorities are hugely underrepresented in higher judiciary. Correct this
-
Remove Raj from Raj Bhawans: Governors are necessary. But as recent controversies like those in Tamil Nadu demonstrate, they must be made accountable to not just the Union but the state and Rajya Sabha as well