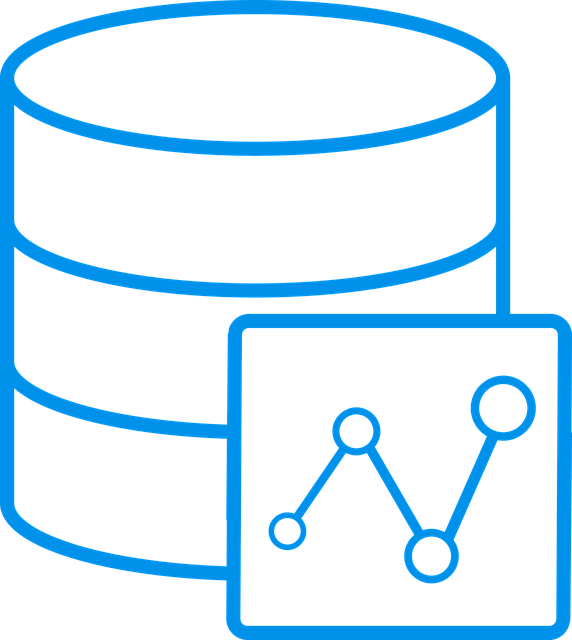
The manufacturing sector faces extraordinary challenges. Among these are: a deep need for modernization; soaring inflation and rising interest rates; energy and fuel bills that may cripple some businesses; looming recession; war in Ukraine; flatlining productivity in some parts of the world; a lack of relevant skills when introducing new technologies; labor shortages due to layoffs, long COVID, and pandemic loss of life; and, of course, global supply-chain disruptions, ranging from scarce or expensive raw materials and transport/logistics challenges to growing cyberattacks on the extended enterprise.
But one company believes that at least some of these challenges, including labor shortages, rising energy costs, and the need for process improvements, can be, if not solved outright, then at least made more manageable via Artificial Intelligence, machine learning, and Digital Twins.
Austin, Texas-based PhD Faustino Gomez is co-founder and CEO of Lugano, Switzerland-headquartered industrial AI company Nnaisense. A specialist in evolutionary enforcement learning – the branch of machine learning in which neural networks work towards incrementally better solutions, discarding unsatisfactory processes in ‘survival of the fittest’ style – Gomez sees a world of potential improvements in manufacturing.
In short, algorithmic natural selection of the best approaches to solving big problems. This week, Nnaisense launched EvoTorch, pitched as “the world’s most advanced evolutionary algorithm library for the machine learning community”, with the ultimate aim of moving beyond human capabilities in many industrial processes.
But claims of easy fixes for the manufacturing sector are often made by vendors. A recent report on Smart Factories by services giant Capgemini found that the reality is often far from simple. Globally, only 14% of such initiatives succeed – due to a dearth of skills, outdated equipment (that was never designed to be networked), the complexity of scaling up pilot programs, and numerous other challenges.
It’s easy to see how a green-field project would be smarter and more efficient from day one, but less so how a long-established factory or brownfield upgrade might be brought into the 2020s without complications.
Automation is at a low level in some countries. For example, the UK is just 24th in the world when it comes to its robot density (the number of industrial machines for every 10,000 human workers), a long way behind industrial rivals in North America, Europe, and Asia. That being the case, how easy is it to push new technology into a sector that may be reluctant to invest?
According to Gomez:
In terms of adoption, resistance, or appetite for doing AI optimization, or adopting AI in general, I see more of a distinction between the UK plus Europe, and the US. I think in the US you see more of an appetite, a recognition that AI is really the way to go for industrial processes, given certain characteristics of those processes.
With the onset of, and the hype surrounding, Industry 4.0 and the IoT, the expectation is that there’s going to be a lot more data, a lot more sensors, so really, the only way to make use of that data, to really leverage it, is to use machine learning.
But the question remains – how easy is it to create a smarter factory out of an established one, rather than a greenfield or pilot implementation? Gomez says:
I think it depends on the scope of what’s intended. But in terms of optimizing existing processes, the fact that it’s a brownfield site doesn’t necessarily interfere, primarily because of the way we do it.
This touches on the larger issue of people not really understanding what machine learning can and can’t do. We view it as an incremental process. You essentially have two kinds of data: static data, which may be the parameters of the setup, the set points for different controls – basically the recipe for the process. And then you have the sensor data that’s collected during the process. Historically, sensor data has had a minimal function of just detecting when things went wrong.
Predictive failure
But now all that data can be used to predict future failures, explains Gomez. Nnaisense is working towards what he calls a “more generic kind of Digital Twin”, in which predictive maintenance and process improvements can be achieved with comparative ease, without always having to build virtual 3D models of physical assets and use them to run simulations. In a factory, a lot of processes are more abstract, but can still be modelled using Nnaisense’s technology, he says:
Since it’s a neural network, it’s learning to predict the future – either the future state of the processes or the quality of the product. We’ve learned directly from sensor data exactly what’s needed.
But for nervous business and IT decision makers, can AI investment in a touch-and-go economy really help them manage challenges like energy usage and supply chain problems? Gomez says:
This touches not just on energy efficiency, but also on the bigger picture of greenhouse gases and global warming. I think it’s really a bottom-up thing as opposed to what Nvidia is talking about [with its global Digital Twin initiative], which is more top down.
You can try to squeeze as much efficiency from your processes as possible and reduce waste, all using traditional methods, but the problem is that many manufacturing processes themselves are becoming more and more complex. By being able to cope with this ‘high dimensional’ data [using our system] and make sense of it, there is significant headroom for improvement.
The system itself learns to optimise processes without necessarily having a lot of domain knowledge. There are processes where it figures out on its own what it needs to do to maximize the reward as specified by the user, which might be reduced energy costs. I think a broad-based, bottom-up approach to reducing greenhouse gas could really help save the planet and make things more efficient.
He adds:
If you use traditional methods, you’re looking at a couple of variables at a time, linear relationships, all that kind of stuff. But with these approaches, you don’t have to do that: the system itself learns what those are in order of achieve the goal.
What it allows you to do, basically, is be better than the teacher. If you look at a system like AlphaGo [Google DeepMind], it’s really learning by imitation, which can only make it as good as the teacher. But EvoTorch allows you to go beyond whatever you might conceive of being the optimal strategy yourself.”
The new system discards solutions that don’t work and constantly moves towards finding better ways of doing things, he says – a process helped by hardware giant NVIDIA’s development of its Isaac robotic simulation tool. He explains:
Isaac allows you to run a full-blown simulation on a GPU, which has allowed us scale EvoTorch up to maybe a million simulations at once, so each of the individual evaluations can be running in parallel across that hardware, which means we can really accelerate the good stuff. You can solve problems quickly and at real scale.
But to what extent can AI and machine learning really help solve labor shortages, other than by simply automating tasks? Gomez argues:
It’s about having robots that are more able to do increasingly complex things and to be more flexible. All of that relies on AI, because it’s really about a robot being an assistant that can perceive the world – function in a closed loop as opposed to pre-programmed trajectories. Something that’s dynamic, and not just a set of rules.
We’re focused on areas where it’s not something that humans can do, or that humans are doing to a certain extent, but the optimal behavior is just not accessible to them.”
My take
A promising and exciting technology with a myriad of applications. But persuading an often-traditional industry to explore solutions that might, to some, appear counter-intuitive, may be Nnaisense’s biggest challenge.
Source: https://diginomica.com/machine-learning-can-help-factories-evolve-says-nnaisense-ceo-faustino-gomez