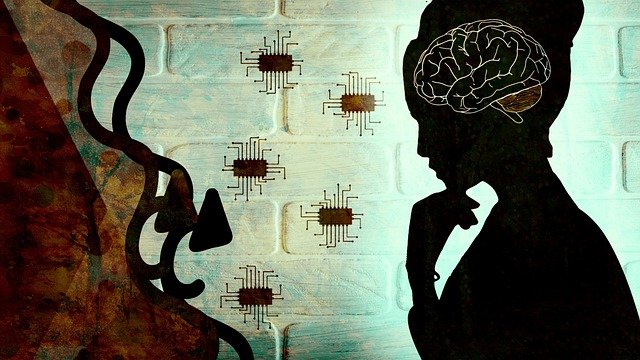
Source: https://arxiv.org/pdf/2106.08245.pdf
Machine Learning has been extensively used in developing accurate interatomic potentials based on initial data of the given chemical system. Atomic Neural Networks(ANN) have proven efficient in building potential functions and describing the local environment. Local environment refers to a part of the whole surroundings that is in contact with system boundaries and is sizable enough to ensure the stability of intensive variables.
Atomic Neural Networks encompasses a set of Machine Learning techniques for forecasting potential energy surfaces and physicochemical properties of materials. In this approach, the total energy is the sum of each atomic energy dependent on the local environment.
The latest developments in machine-learned interatomic potentials are based on the atomistic representation and locally invariant many-body descriptors. However, it has been established that three-body or four-body descriptors could stir local structural degeneracy and thus fail to describe the local environment completely.
An alternative approach would be to repeatedly convolute feature vectors between every atom and its neighbors by neural networks that allow the intelligence to pass on from the central atom to its neighbors. This method, called Message Passing Neural Network(MPNN), can learn an increasingly sophisticated feature-property correlation. Nevertheless, there is little clarity on how the model can resolve the issue of local structural degeneracy and effectively embrace many-body correlations by iteratively integrating two-body terms and angular terms.
To tackle this limitation, a team of researchers from the University of Science and Technology of China (USTC) has proposed a Recursively Embedded Atom Neural Network (REANN) model. Their study provides formal proof concerning the model’s efficiency in incorporating many-body correlations without explicitly putting a figure on high-order terms.
Source: https://arxiv.org/pdf/2106.08245.pdf
The proposed model naturally integrates the message-passing concept into a well-defined three-body descriptor. The model proclaims to create a full-fledged atomic representation of the local environment and include a few non-local interactions validated by numerical tests.
The REANN package is implemented in PyTorch, which allows for highly productive optimization of all parameters. All the tuning parameters, including α and orbital coefficients, were optimized along with the weights and biases of neural networks (NNs) to reduce the cost function using the mini-batch gradients algorithm.
By testing the Methane(CH4) and bulk water datasets, the researchers demonstrated the local completeness and nonlocality of the REANN model. The CH4 dataset was curated for checking the manifestation of the structural descriptors that comprise near-degenerate configurations with clearly defined energies and meta-physical configurations. On the other hand, bulk water was cleaved into two independent datasets to exemplify the performance of the new REANN model.
By analyzing the two datasets, the study reveals the increased accuracy and coherency of the REANN model. The motive of the study is to provide a way to effortlessly enhance existing ML potential surface frameworks to include more complicated many-body descriptors without modifying their primary structures.
Paper: https://arxiv.org/pdf/2106.08245.pdf
Github: https://github.com/zhangylch/Physically-motivated-Recursively-Embedded-Atom-Neural-Networks
References:
- https://phys.org/news/2021-10-recursively-embedded-atom-neural-network.html
Suggested
RELATED ARTICLESMORE FROM AUTHOR